Domain Adaptation Generative Adversarial Networks
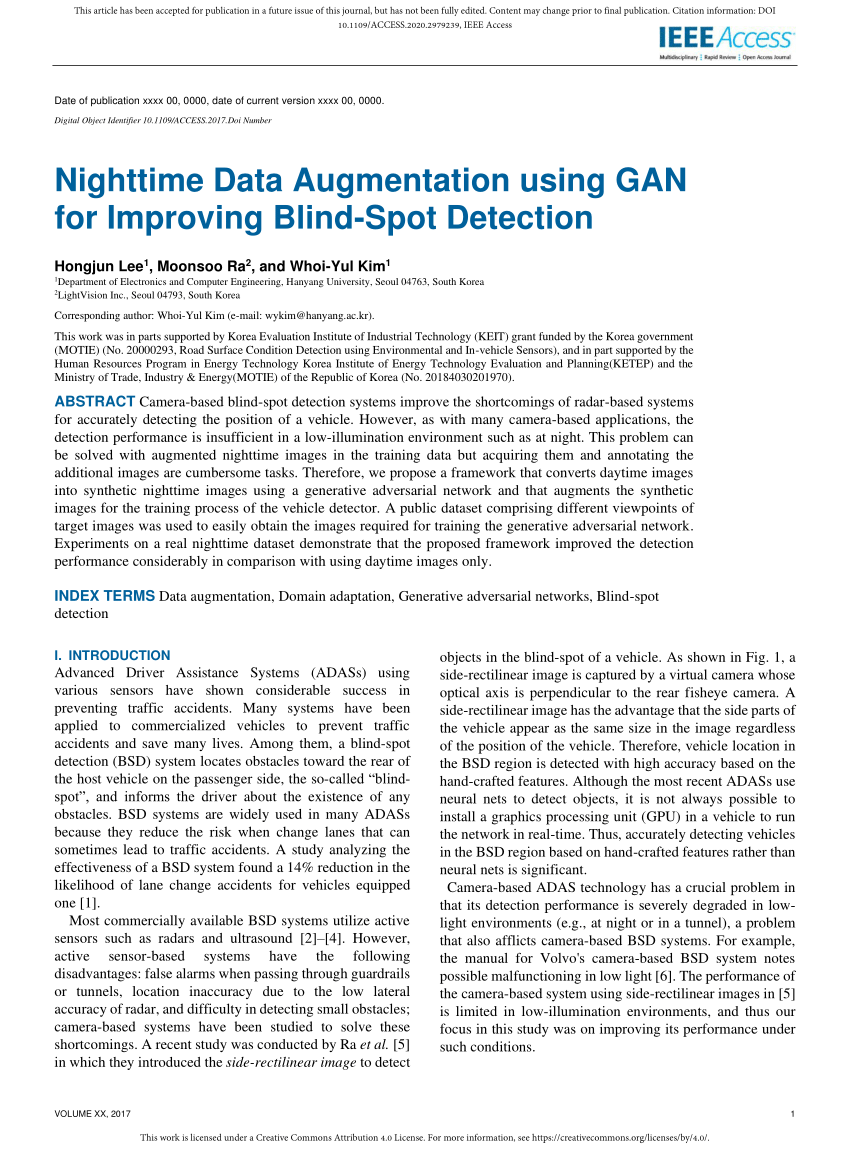
Adversarial multiple source domain adaptation.
Domain adaptation generative adversarial networks. Rau a 1 edwards pje 2 ahmad of 2 riordan p 3 janatka m 2 lovat lb 2 stoyanov d 2. 107109 10 1016 j patcog 2019 107109 article download pdf google scholar. Cross dataset facial emotion recognition fer aims to reduce the discrepancy between the source and the target facial database.
Unsupervised domain adaptation using generative adversarial networks for semantic segmentation of aerial images pdf available via license. Cc by 4 0 content may be subject to copyright. Naturally leads to an efficient learning strategy using adversarial neural networks.
Getraining data independent image registration using generative adversarial networks and domain adaptation pattern recognit 100 2020 p. Unsupervised pixel level domain adaptation with generative adversarial networks.
In this work we propose a novel generative adversarial network gan namely videogan to transfer the video based data across different domains. In this work we propose an approach. Castillo rama chellappa umiacs university of maryland college park abstract domain adaptation is an actively researched problem in computer vision.
Current unsupervised domain adaptation uda methods based on gan generative adversarial network architectures assume that source samples arise from a single distribution. These methods have shown compelling results by finding the transformation between source and target domains to reduce the distribution divergence. Implicit domain adaptation with conditional generative adversarial networks for depth prediction in endoscopy.
Aligning domains using generative adversarial networks swami sankaranarayanan yogesh balaji carlos d. Cross dataset facial emotion recognition fer aims to reduce the discrepancy between the source and the target facial database. We show how to interpret it as learning feature representations that are invariant to the multiple domain shifts while still being discriminative for the learning task.