Domain Adaptation Of Learned Features For Visual Localization
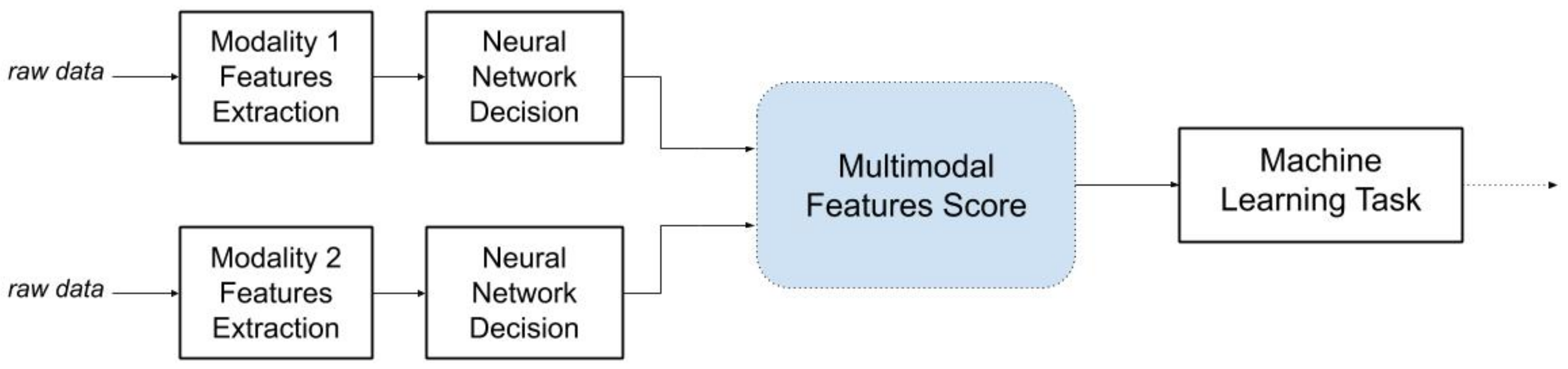
Unlike in inductive transfer where.
Domain adaptation of learned features for visual localization. Recent learned local features based on deep neural networks have shown superior performance over classical hand crafted local features. The localization strategy. However sfm itself relies on local features which are prone.
2 1 domain adaptation we now formalize the problem of domain adaptation. In multi source domain adaptation f is learned from more than one domain in saccompanying each of the above two cases. A domain is a distribution d on the instance set x.
The aim of this paper is to give an overview of domain adaptation and transfer learning with a specific view on visual applications. After a general motivation we first position domain adaptation. Second we try to address and analyze briefly the state of the art methods for different types of scenarios first describing the historical shallow methods.
High quality datasets with accurate 6 degree of freedom dof reference poses are the foundation for benchmarking and improving existing methods. To avoid confusion we will always mean a speciļ¬c distribution over the instance set when we say domain. Recent learned local features based on deep neural networks have shown superior performance over classical hand crafted local features.
08 21 2020 by sungyong baik et al. The aim of this paper is to give an overview of domain adaptation and transfer learning with a specific view on visual applications. However in a real world scenario there often exists a large domain gap between training and target images which can significantly degrade the.
In unsupervised domain adaptation the function f is learned using the knowledge in sand t u. 0 share. Domain adaptation of learned features for visual localization we tackle the problem of visual localization under changing conditions such as time of day weather and seasons.