Domain Adaptation Using Reinforcement Learning
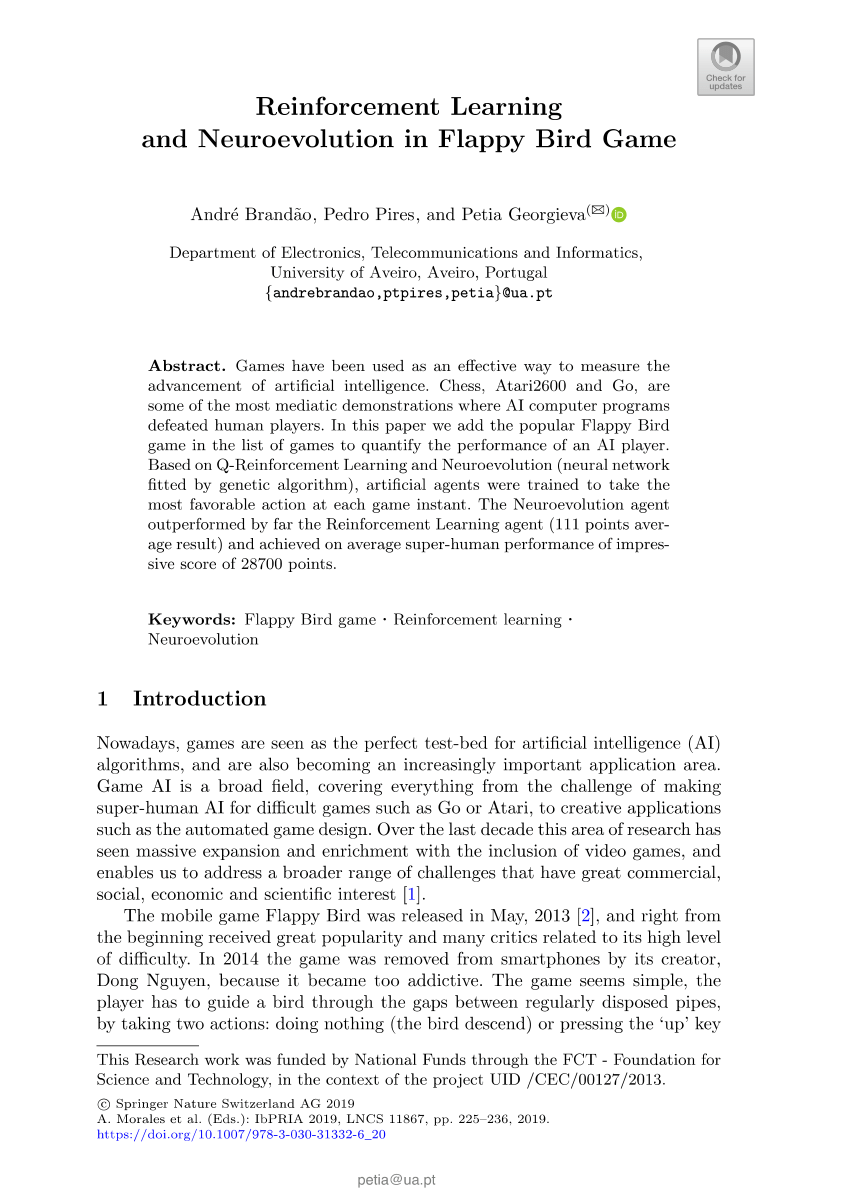
Many deep reinforcement learning approaches use graphical state representations.
Domain adaptation using reinforcement learning. Of the 18th international conference on autonomous agents and multiagent systems aamas 2019 montreal canada may 13 17 2019 ifaamas 3 pages. Using gaussian process based reinforcement learning it has been shown that it is possible to construct generic policies which provide acceptable in domain user performance and better performance than can be obtained using under trained domain specific policies. Reinforcement learning can be understood using the concepts of agents environments states actions and rewards all of which we ll explain below.
Domain adaptation for reinforcement learning on the atari. 1 introduction deep reinforcement learning drl successfully extends the re. This issue magnifies when facing continuous domains where the curse of dimensionality is inevitable and generalization is mostly desired.
The goal of a domain adaptation approach is to learn and find trans formations which can map both source and target domains into a common feature space. To adaptively learn data values jointly with the target task predictor model we propose a meta learning framework which we name data valuation using reinforcement learning dvrl. Partial domain adaptation aims to transfer knowledge from a label rich source domain to a label scarce target domain which relaxes the fully shared label space assumption across different domains.
In this more general and practical scenario a major challenge is how to select source instances in the shared classes across different domains for positive transfer. On the other hand. Learning to transfer examples for partial domain adaptation.
To address this issue we. Capital letters tend to denote sets of things and lower case letters denote a specific instance of that thing. 1 building insights about the learning task 2 domain adaptation 3 corrupted sample discovery and 4 robust learning.
Domain adaptation is a well known technique associated with transfer learning which seeks the same goal in machine learning problems especially pattern recognition. Data valuation has multiple important use cases. Unsupervised domain adaptation using deep networks with cross grafted stacks arxiv 17 feb 2019.