Domain Generalization Deep Learning
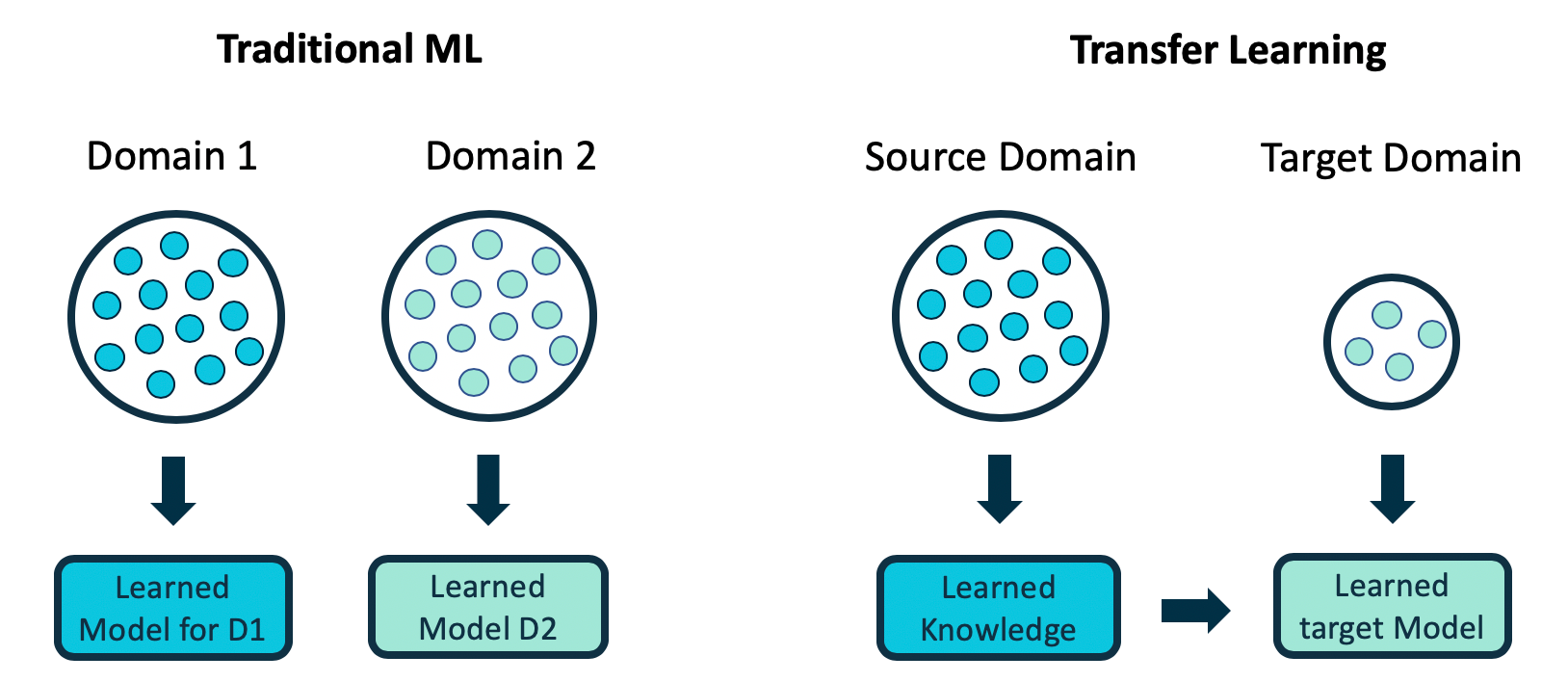
In this paper we propose adversarial domain augmenta tion sec.
Domain generalization deep learning. In this paper we propose a sequential learning framework for domain generalization dg the problem of training a model that is robust to domain shift by design. 2 generalization and capacity control in deep learning in this section we discuss complexity measures that have been suggested or could be used for capacity control in neural networks. Sequential learning for domain generalization.
University of surrey 0 share. This paper proposes a deep learning based domain generalization method for rotating machinery fault diagnosis. The idea of domain generalization is to learn from one or multiple training domains to extract a domain agnostic model which can be applied to an unseen domain.
Learning paradigm for single domain generalization. Domain generalization attempts to learn a domain invariant representation given input data from multiple domains 25. Kot1 1school of electrical and electronic engineering nanyang technological university singapore 2school of computer science and engineering nanyang technological university singapore 3department of computer science city university of hong kong china.
The dataset folder path save root. However unlike domain adaptation in domain generalization target domain is not available during training. 3 1 to solve this challenging task.
Of domain generalization using a novel regularization function. If you want to train the model without domain generalization deep all you can also use the following command. Our pacs photo art cartoon sketch dataset is aligned with a practical application of domain generalization and we showed it has more challenging domain shift than prior datasets making it suitable to drive the field in future.
The goal is to use sin. The gpu id to run experiments. We pose the problem of finding such a regularization function in a learning to learn or meta learning framework.